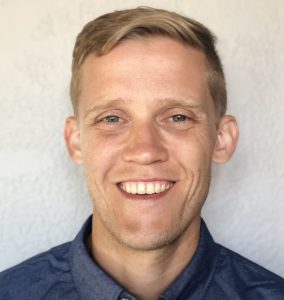
Mason Earles is Assistant Professor of Biological & Agricultural Engineering and Viticulture & Enology at UC Davis, along with a Co-PI on the National AI Institute for Next Generation Food Systems. He worked at Apple Inc. on machine learning and computer vision applications within hardware engineering for several years, and prior to that he developed novel methods in computational and experimental plant physiology. He currently leads the Plant AI and Biophysics Lab at UC Davis which aims to develop AI-enabled sensing solutions for agriculture and food systems.
Despite the existence of at least 50+ public agricultural ML datasets, they are distributed across numerous servers, maintained at various levels, and stored in a wide variety of formats. This lack of centralization and standardization is a major barrier for data reusability, benchmarking, and model pre-training. Second, “out-of-the-box” model architectures and pipelines are often suboptimal for many agricultural AI problems, creating a need for accessible agriculture-specific model building tools. Third, despite substantial development in 3D digital crop modeling9, no common framework exists for synthetic training data generation in agricultural crops. To overcome these challenges there is a need to develop infrastructure to accelerate the scaling of agricultural AI technologies. In this talk, we introduce an open-source Python framework, called AgML, which aims to enable access to agricultural-specific machine learning (ML) datasets, benchmarks, pre-trained models, workflows, and synthetic data generators. We invite collaborators to join the AgML community at https://github.com/Project-AgML.